Neurocybernetic Assistive Technologies to Enhance Robotic Wheelchair Navigation
Poly Rani Ghosh 1*, Maria Afnan 1, Joy Biswas 1
Journal of Primeasia 4(1) 1-6 https://doi.org/10.25163/primeasia.4140044
Submitted: 13 February 2023 Revised: 17 April 2023 Published: 19 April 2023
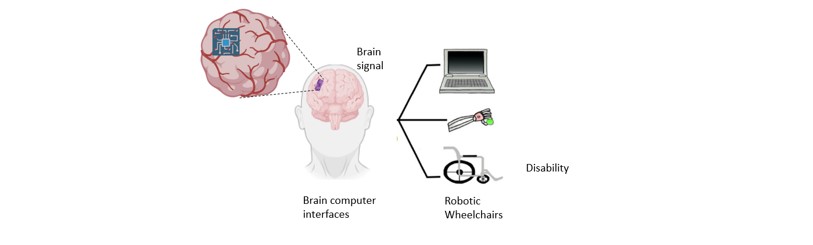
Abstract
Millions of individuals worldwide face mobility impairments and rely on wheelchairs for assistance. Yet a significant portion of individuals with severe motor disabilities or insufficient familiarity with conventional interfaces cannot consider powered wheelchairs as a viable solution. Neurocybernetics present a promising solution to this challenge. Neurocybernetics involves exploring how the nervous system interacts with systems, like computers and robots. Through the use of interfaces users can control wheelchairs using neural signals from their brains making navigation more intuitive and effective. Assistive technologies, especially robotic wheelchairs powered by sensors and control systems, are essential for those disabled people, with mobility issues, to live more independently and better lives in their daily life even without the assistance of others. The primary objective of this study is to improve the quality of life for individuals with disabilities who depend on such assistance for their mobility requirements. This article examines some of the cutting-edge neurocybernetic devices that assist people with disabilities, such as brain-computer interfaces (BCIs), algorithms to understand brain signals, and adaptive control methods for wheelchairs. This study proposes a robotic wheelchair which utilizes the human brain signal and undergoes machine learning based classification model with neuromorphic adaptive control. It discusses the key considerations, difficulties, and potential uses of neurocybernetic robotic wheelchairs in real life situations. Additionally, it addresses the ethical, legal, and societal implications of using these technologies and suggests future research areas to advance their creation and use.
Keywords: Neurocybernetics, Robotic Wheelchairs. Brain-Computer Interfaces, Assistive Technologies, Mobility Impairments.
References
ATA, A. M. B. (2021). Autoregression features for smart robotic wheelchair EEG-ICA classification using a bagging model.
Benevides, A. B., Bastos, T. F., & Sarcinelli Filho, M. (2011, June). Proposal of brain-computer interface architecture to command a robotic wheelchair. In 2011 IEEE International Symposium on Industrial Electronics (pp. 2249-2254). IEEE.
Candiotti, J. L., Daveler, B. J., Kamaraj, D. C., Chung, C. S., Cooper, R., Grindle, G. G., & Cooper, R. A. (2019). A heuristic approach to overcome architectural barriers using a robotic wheelchair. IEEE Transactions on Neural Systems and Rehabilitation Engineering, 27(9), 1846-1854.
Carlson, T., & Demiris, Y. (2012). Collaborative control for a robotic wheelchair: Evaluation of performance, attention, and workload. IEEE Transactions on Systems, Man, and Cybernetics, Part B (Cybernetics), 42(3), 876-888.
Chen, X., Wang, Y., Nakanishi, M., Gao, X., Jung, T. P., & Gao, S. (2015). High-speed spelling with a noninvasive brain–computer interface. Proceedings of the National Academy of Sciences, 112(44), E6058-E6067.
de Almeida Afonso, P., & Ferreira Jr, P. R. (2023). Autonomous navigation of wheelchairs in indoor environments using deep reinforcement learning and computer vision.
Del Castillo, G., Skaar, S., Cardenas, A., & Fehr, L. (2006). A sonar approach to obstacle detection for a vision-based autonomous wheelchair. Robotics and Autonomous Systems, 54(12), 967-981.
Delmas, S., Morbidi, F., Caron, G., Albrand, J., Jeanne-Rose, M., Devigne, L., & Babel, M. (2021, January). SpheriCol: A driving assistance system for power wheelchairs based on spherical vision and range measurements. In 2021 IEEE/SICE International Symposium on System Integration (SII) (pp. 505-510). IEEE.
Delmas, S., Morbidi, F., Caron, G., Babel, M., & Pasteau, F. (2023). SpheriCol: A driving assistant for power wheelchairs based on spherical vision. IEEE Transactions on Medical Robotics and Bionics.
Devigne, L., Pasteau, F., Babel, M., Narayanan, V. K., Guegan, S., & Gallien, P. (2018, October). Design of a haptic guidance solution for assisted power wheelchair navigation. In 2018 IEEE International Conference on Systems, Man, and Cybernetics (SMC) (pp. 3231-3236). IEEE.
Erdogan, A., & Argall, B. D. (2017). The effect of robotic wheelchair control paradigm and interface on user performance, effort and preference: An experimental assessment. Robotics and Autonomous Systems, 94, 282-297.
Ezeh, C., Trautman, P., Devigne, L., Bureau, V., Babel, M., & Carlson, T. (2017, July). Probabilistic vs linear blending approaches to shared control for wheelchair driving. In 2017 International Conference on Rehabilitation Robotics (ICORR) (pp. 835-840). IEEE.
Ghezala, A. A., Sentouh, C., & Pudlo, P. (2022). Direct model-reference adaptive control for wheelchair simulator control via a haptic interface. IFAC-PapersOnLine, 55(29), 49-54.
How, T. V., Wang, R. H., & Mihailidis, A. (2013). Evaluation of an intelligent wheelchair system for older adults with cognitive impairments. Journal of NeuroEngineering and Rehabilitation, 10, 1-16.
Ikeda, H., Toyama, T., Maki, D., Sato, K., & Nakano, E. (2021). Cooperative step-climbing strategy using an autonomous wheelchair and a robot. Robotics and Autonomous Systems, 135, 103670.
Jeong, W., Kwon, M., Youm, K., Jeon, H., & Oh, S. (2024). Design of Wheelchair Drive Unit Capable of Driving on Roads and Obstacles with Shape Conversion. Applied Sciences, 14(4), 1434.
Jin, J., Zhang, H., Daly, I., Wang, X., & Cichocki, A. (2017). An improved P300 pattern in BCI to catch user’s attention. Journal of Neural Engineering, 14(3), 036001.
Kutbi, M., Du, X., Chang, Y., Sun, B., Agadakos, N., Li, H., ... & Mordohai, P. (2020). Usability studies of an egocentric vision-based robotic wheelchair. ACM Transactions on Human-Robot Interaction (THRI), 10(1), 1-23.
LaFleur, K., Cassady, K., Doud, A., Shades, K., Rogin, E., & He, B. (2013). Quadcopter control in three-dimensional space using a noninvasive motor imagery-based brain–computer interface. Journal of Neural Engineering, 10(4), 046003.
Leblong, E., Fraudet, B., Devigne, L., Babel, M., Pasteau, F., Nicolas, B., & Gallien, P. (2021). SWADAPT1: Assessment of an electric wheelchair-driving robotic module in standardized circuits: A prospective, controlled repeated measure design pilot study. Journal of NeuroEngineering and Rehabilitation, 18, 1-12.
Li, H., Yuan, D., Ma, X., Cui, D., & Cao, L. (2017). Genetic algorithm for the optimization of features and neural networks in ECG signals classification. Scientific Reports, 7(1), 41011.
Morales, Y., Watanabe, A., Ferreri, F., Even, J., Shinozawa, K., & Hagita, N. (2018). Passenger discomfort map for autonomous navigation in a robotic wheelchair. Robotics and Autonomous Systems, 103, 13-26.
Morbidi, F., Devigne, L., Teodorescu, C. S., Fraudet, B., Leblong, E., Carlson, T., & Ragot, N. (2022). Assistive robotic technologies for next-generation smart wheelchairs: Codesign and modularity to improve users’ quality of life. IEEE Robotics & Automation Magazine, 30(1), 24-35.
Ngo, B. V., & Nguyen, T. H. (2022). A semi-automatic wheelchair with navigation based on virtual-real 2D grid maps and EEG signals. Applied Sciences, 12(17), 8880.
Podobnik, J., Rejc, J., Slajpah, S., Munih, M., & Mihelj, M. (2017). All-terrain wheelchair: Increasing personal mobility with a powered wheel-track hybrid wheelchair. IEEE Robotics & Automation Magazine, 24(4), 26-36.
Rebsamen, B., Guan, C., Zhang, H., Wang, C., Teo, C., Ang, M. H., & Burdet, E. (2010). A brain controlled wheelchair to navigate in familiar environments. IEEE Transactions on Neural Systems and Rehabilitation Engineering, 18(6), 590-598.
Reddy, A. G., & Narava, S. (2013). Artifact removal from EEG signals. International Journal of Computer Applications, 77(13).
Simpson, R. C., LoPresti, E. F., & Cooper, R. A. (2008). How many people would benefit from a smart wheelchair? Journal of Rehabilitation Research & Development, 45(1).
Sinyukov, D., Desmond, R., Dickerman, M., Fleming, J., Schaufeld, J., & Padir, T. (2014). Multi-modal control framework for a semi-autonomous wheelchair using modular sensor designs. Intelligent Service Robotics, 7, 145-155.
Sumikura, S., Shibuya, M., & Sakurada, K. (2019, October). OpenVSLAM: A versatile visual SLAM framework. In Proceedings of the 27th ACM International Conference on Multimedia (pp. 2292-2295).
Teodorescu, C. S., Caplan, I., Eberle, H., & Carlson, T. (2021, June). Model-based sensor fusion and filtering for localization of a semi-autonomous robotic vehicle. In 2021 European Control Conference (ECC) (pp. 1283-1290). IEEE.
Vailland, G., Devigne, L., Pasteau, F., Nouviale, F., Fraudet, B., Leblong, E., & Gouranton, V. (2021, March). VR based power wheelchair simulator: Usability evaluation through a clinically validated task with regular users. In 2021 IEEE Virtual Reality and 3D User Interfaces (VR) (pp. 420-427). IEEE.
Viswanathan, P., Zambalde, E. P., Foley, G., Graham, J. L., Wang, R. H., Adhikari, B., ... & Mitchell, I. M. (2017). Intelligent wheelchair control strategies for older adults with cognitive impairment: User attitudes, needs, and preferences. Autonomous Robots, 41, 539-554.
Wolpaw, J. R., Birbaumer, N., Heetderks, W. J., McFarland, D. J., Peckham, P. H., Schalk, G., ... & Vaughan, T. M. (2000). Brain-computer interface technology: A review of the first international meeting. IEEE Transactions on Rehabilitation Engineering, 8(2), 164-173.
Wolpaw, J. R., Birbaumer, N., McFarland, D. J., Pfurtscheller, G., & Vaughan, T. M. (2002). Brain–computer interfaces for communication and control. Clinical Neurophysiology, 113(6), 767-791).
World Health Organization: WHO. (2024, January 2). Assistive technology. Retrieved from https://www.who.int/news-room/fact-sheets/detail/assistive-technology
Wu, B. F., Chen, Y. S., Huang, C. W., & Chang, P. J. (2018). An uphill safety controller with deep learning-based ramp detection for intelligent wheelchairs. IEEE Access, 6, 28356-28371.
Zolotas, M., Elsdon, J., & Demiris, Y. (2018, October). Head-mounted augmented reality for explainable robotic wheelchair assistance. In 2018 IEEE/RSJ International Conference on Intelligent Robots and Systems (IROS) (pp. 1823-1829). IEEE.
View Dimensions
View Altmetric
Save
Citation
View
Share